The Web is evolving through an era where the opinions of users are getting increasingly important and valuable. The distillation of knowledge from the huge amount of unstructured information on the Web can be a key factor for tasks such as social media marketing, branding, product positioning, and corporate reputation management. These online social data, however, remain hardly accessible to computers, as they are specifically meant for human consumption. The automatic analysis of online opinions involves a deep understanding of natural language text by machines, from which we are still very far.
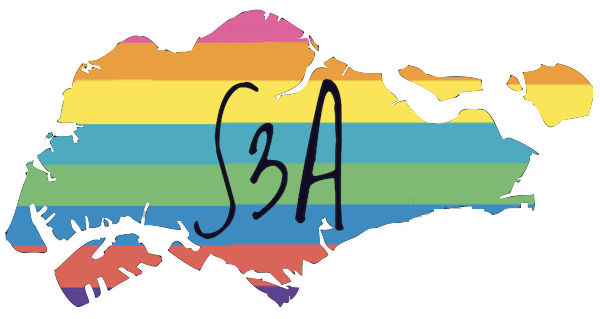
Singapore Symposium on Sentiment Analysis (S3A) is a biennial event aiming to bridge such a gap by exploring novel approaches to opinion mining and sentiment analysis that enable a more efficient passage from (unstructured) textual information to (structured) machine-processable data. S3A aims to provide a national forum for Singapore-based researchers working in the field of sentiment analysis and related topics to share information on their latest investigations and their applications both in academic research areas and industrial sectors.
• S3A'25 (27th February 2025, NTU)
• S3A'23 (9th March 2023, NTU)
• S3A'21 (2nd March 2021, virtual)
• S3A'19 (8th March 2019, PARKROYAL)
• S3A'17 (17th February 2017, NTU)
• S3A'15 (6th February 2015, NTU)
• S3A'13 (1st November 2013, NTU)
The broader context of the symposium comprehends AI, linguistics, psychology, sociology, and ethics. Topics of interest include but are not limited to:
• Sentiment identification & classification
• Opinion and sentiment summarization & visualization
• Social network analysis
• Social media marketing
• Cultural-dependent sentiment analysis
• Personality detection
• Aspect extraction for opinion mining
• Linguistic patterns for sentiment analysis
• Learning word dependencies in text
• Statistical learning theory for big social data analysis
• Deep learning for sarcasm detection
• Sentic computing
• Large commonsense graphs
• Conceptual primitives for sentiment analysis
• Multimodal emotion recognition and sentiment analysis
• Multi-domain & cross-domain evaluation
• Opinion spam detection
S3A'25 (27th February 2025, NTU)
S3A hosts some of the best Singapore-based sentiment analysis researchers. This year, the event will take place at NTU LT27 on 27th February from 11.20am to 1.20pm Singapore time. Attendance is free of charge but registration is mandatory: REGISTRATION LINK (if you have already registered for any of the previous editions, you do not need to register again). Besides food for thought, coffee/tea and a buffet lunch will be provided. The format of the event consists of consecutive 10-min presentations followed by 5-min Q&A sessions. For enquires regarding the event, please contact s3a@sentic.net. Stay tuned and see you soon!
11.20 – 11.30: Welcoming and introduction
11.30 – 11.45: Donghao Huang (Mastercard)
Chinese Sentiment Analysis: Subjects, Methods, and TrendsSentiment analysis is a key area in natural language processing, with growing research interest. While many reviews cover sentiment analysis techniques and applications, there is limited focus on thematic trends and research methodologies, especially for Chinese text. This study fills that gap by surveying research topics, methods, and trends in Chinese sentiment analysis. Using keyword co-occurrence analysis and community detection, it provides a fresh perspective on the field. By examining methodological and thematic developments over two decades, the study highlights key trends, emerging topics, and research gaps, offering valuable insights for academics and practitioners.
11.45 – 12.00: Xulang Zhang (NTU CCDS)
Discovering the Variations of Lexical Semantics between Languages
Multilingual emotion recognition is crucial for cross-cultural communication and data mining. While past research has focused on accuracy, it often ignores semantic disparities across languages. This study addresses this gap by identifying lexical semantic variations and integrating them into a multilingual emotion recognition model. Since pre-trained models are biased toward English, they misinterpret emotions in other languages. Our semantic variation injection method mitigates this issue, improving accuracy. These findings advance culturally sensitive emotion recognition and provide insights for linguistics and computational linguistics in multilingual research.
12.00 – 12.15: Gianmarco Mengaldo (National University of Singapore)
Explainable NLP for Corporate Sustainability Analysis
Sustainability refers to individuals, companies, and institutions minimizing harm—or even benefiting—the environment, society, and the economy. As it becomes synonymous with acceptable behavior, sustainability is increasingly regulated, with various frameworks, such as the UN’s sustainable development goals and global reporting standards, assessing corporate impact. However, corporate sustainability is complex due to factors like geography, size, and stakeholder interconnections. Assessments are plagued by subjectivity in both corporate disclosures and analyst evaluations, leading to challenges like data incompleteness, ambiguity, unreliability, and bias. This weakens accountability and regulatory effectiveness. Explainable NLP (XNLP) can enhance analysis by integrating linguistic understanding with explainability, improving resource efficiency and reducing subjectivity.
12.15 – 12.30: Minne Chen (NTU SSS)
Understanding Sentiments Around ChildbearingSentiment analysis and computational methods are increasingly utilized in the social sciences. This presentation will explore some recent applications of these methods, highlighting current trends, the potential of sentiment analysis, and the challenges researchers encounter in the discipline of Sociology. In addition, I will introduce an early-stage study that focuses on analyzing sentiments surrounding decisions to parenthood. This study examines the complex emotions influencing individuals' decisions about parenthood, utilizing sentiment analysis, sociological insights, and qualitative narratives to delve into the interplay of mixed feelings, including joy, anxiety, and societal pressures. We will discuss our data cleaning strategies, emphasizing the challenges of processing extensive datasets effectively for very specific research questions within social science.
12.30 – 12.45: Rui Mao (NTU CCDS)
Metaphor and Cognition: Our Unconscious Emotional Expressions
Metaphors, beyond their linguistic function, offer insights into underlying cognitive structures through their associated concept mappings and emotional resonance. This presentation introduces a computational tool for analysing metaphors and investigates the interplay between metaphorical language, neural activity, and behaviour. We will explore how metaphorical expressions in diverse domains, including technology, mental health, and politics, reveal underlying cognitive patterns and offer insights into how we conceptualize these complex areas of human experience.
12.45 – 13.00: Deeksha Varshney (National University of Singapore)
Sentiment Analysis on Climate Change for Sustainable Investment
This research examines whether climate change sentiment is a systematic risk factor in sustainable finance. Tweets from 2014–2022 are collected via the Twitter API, with RoBERTa labeling sentiment polarity and DistilBERT classifying topics (Aggregated, Impact, Politics and Policy, Mitigation, Root Cause). Monthly sentiment scores are derived from sentiment frequency and categorized by topic. Simultaneously, monthly returns of green ETFs, sector ETFs (technology, oil and gas), and stock baskets (sustainable vs. heavy-polluting) are collected. Fama-French regressions are then applied, incorporating sentiment scores alongside traditional risk factors.
13.00 – 13.15: Erik Cambria (NTU CCDS)
Commonsense AI for Interpretable, Trustworthy, and Explainable Affective ComputingWhile ChatGPT and DeepSeek have unlocked solutions to problems that were previously considered unsolvable, they also introduced new ones. One of such problems is the phenomenon known as hallucination, the generation of content that is nonsensical or unfaithful to the provided source content. SenticNet 8, a neurosymbolic AI framework leveraging an ensemble of commonsense knowledge representation and hierarchical attention networks, which aims to mitigate some of these issues in the context of affective computing. In particular, we focus on the tasks of sentiment analysis, personality prediction, and suicidal ideation detection. Results show that SenticNet 8 presents superior accuracy with respect to all four baselines, namely: bag-of-words, word2vec, RoBERTa, and ChatGPT.
13.15 – 13.20: Summary and concluding remarks
S3A'23 (9th March 2023, NTU)
10.30 – 10.35: Welcoming and introduction
10.35 – 11.00: Wenxuan Zhang (DAMO Academy, Alibaba Group)
A Survey on Aspect-Based Sentiment Analysis: Tasks, Methods, and Challenges
Unlike early aspect-based sentiment analysis (ABSA) works focusing on a single sentiment element, many compound ABSA tasks involving multiple elements have also been studied in recent years for capturing more complete aspect-level sentiment information. In this survey, we provide a new taxonomy for ABSA which organizes existing studies from the axes of concerned sentiment elements, with an emphasis on recent advances of compound ABSA tasks. We also summarize the utilization of pre-trained language models for ABSA, which improved the performance of ABSA to a new stage. Finally, we review some emerging topics and discuss some open challenges to outlook potential future directions of ABSA.
11.00 – 11.15: Lizi Liao (SMU School of Computing and Information Systems)
DiaASQ: A Benchmark of Conversational Aspect-based Sentiment Quadruple Analysis
The rapid development of ABSA within recent decades shows great potential for real-world society. The current ABSA works, however, are mostly limited to the scenario of a single text piece, leaving the study in dialogue contexts unexplored. In this work, we introduce a novel task of conversational aspect-based sentiment quadruple analysis, namely DiaASQ, aiming to detect the sentiment quadruple of target-aspect-opinion-sentiment in a dialogue. DiaASQ bridges the gap between fine-grained sentiment analysis and conversational opinion mining. We manually construct a large-scale, high-quality Chinese dataset and also obtain the English version dataset via manual translation.
11.15 – 11.30: Kelvin Du (Vice President, Data Science, DBS Bank)
Incorporating Knowledge Sources for Targeted Aspect-based Financial Sentiment Analysis
Combining symbolic and subsymbolic methods has become a promising strategy as research tasks in AI grow increasingly complicated and require higher levels of understanding. Targeted Aspect-based Financial Sentiment Analysis (TABFSA) is an example of such complicated tasks, as it involves processes like information extraction, information speciication, and domain adaptation. However, little is known about the design principles of such hybrid models leveraging external lexical knowledge. To ill this gap, we deine anterior, parallel, and posterior knowledge integration and propose incorporating multiple lexical knowledge sources strategically into the ine-tuning process of pre-trained transformer models for TABFSA.
11.30 – 11.45: Keane Ong Wei Yang (NUS Asian Institute of Digital Finance)
Explainable Finance through Aspect-Based Sentiment Analysis
This study presents a novel approach for explainability in financial analysis by utilizing the Pearson correlation coefficient to establish a relationship between aspect-based sentiment analysis and stock prices. The proposed methodology involves constructing an aspect list from financial news articles and analyzing sentiment intensity scores for each aspect. These scores are then compared to the stock prices for the relevant companies using the Pearson coefficient to determine any significant correlations.
11.45 – 12.00: Wei Li (NTU School of Computer Science and Engineering)
SKIER: A Symbolic Knowledge Integrated Model for Conversational Emotion Recognition
Emotion recognition in conversation (ERC) has received increasing attention from the research community. However, it is a challenging task, largely due to the complex and unstructured properties of multi-party conversations. In this work, we address these challenges by explicitly modeling the discourse relations between utterances and incorporating symbolic knowledge into multi-party conversations. We first introduce a dialogue parsing algorithm into ERC and further improve the algorithm through a transfer learning method. Moreover, we leverage different symbolic knowledge graph relations to learn knowledge-enhanced features for the ERC task.
12.00 – 12.15: Cuc Duong (NTU Interdisciplinary Graduate School)
ClimateTweets: Saving Earth One Tweet at a Time through the Lens of AI
The impacts of climate change and global warming have become more visible globally because of the increasing frequency of extreme weather events, abnormal heatwaves, and other climate crises. Besides the traditional survey method, it is beneficial to automatically distillate climate change opinions from social platforms to measure public reactions quickly. We investigate how to organize climate change opinions on Twitter into meaningful categories to support perspective summarizing tasks. We find that merely using the available taxonomy for this task is ineffective; hence we consider the entire text content. We recommend five high-level categories (Root cause, Impact, Mitigation, Politics or Policy, Others) and assemble ClimateTweets, a dataset with category and polarity labels.
12.15 – 12.30: Vlad Pandelea (Continental-NTU Corporate Lab)
Software-Hardware Co-Design for Sentiment AnalysisWe propose an architecture search inspired by Neural Architecture Search methods. The search is driven by the concept of software-hardware co-design at application level, that is new in sentiment analysis. This task is of particular interest for multiple reasons. First, sentiment analysis state-of-the-art solutions are known to rely on large pre-trained models, which are finetuned to maximize performance, but strategies on how to fully utilize their feature extraction capabilities without fine-tuning are understudied. Moreover, sentiment is user-specific to a great extent and thus being able to deploy customized solutions is an important but overlooked aspect. Little research in this area has focused on integrating hardware constraints into solution design.
12.30 – 12.45: Zhaoxia Wang (SMU School of Computing and Information Systems)
Mimicking Human Language Understanding for Fine-grained Multi-class Sentiment AnalysisThis work proposes a new explainable fine-grained multi-class sentiment analysis method, namely MiMuSA, which mimics the human language understanding processes. The proposed method involves a multi-level modular structure designed to mimic human’s language understanding processes, e.g., ambivalence handling process, sentiment strength handling process, etc. Specifically, multiple knowledge bases including basic knowledge base, negation and special knowledge base, sarcasm rule and adversative knowledge base, and sentiment strength knowledge base are built to support the sentiment understanding process.
12.45 – 13.00: Erik Cambria (NTU School of Computer Science and Engineering)
SenticNet 7: A Neurosymbolic AI Framework for Explainable Sentiment AnalysisThe key limitations of current AI models are: dependency, reproducibility, trustworthiness, interpretability, and explainability. In this work, we propose a commonsense-based neurosymbolic framework that aims to overcome these issues in the context of sentiment analysis. In particular, we employ unsupervised and reproducible subsymbolic techniques such as auto-regressive language models and kernel methods to build trustworthy symbolic representations that convert natural language to a sort of protolanguage and, hence, extract polarity from text in a completely interpretable and explainable manner.
13.00 – 13.15: Björn W. Schuller (University of Augsburg, Imperial College London)
Will Affective Computing Emerge from Foundation Models and General AI?ChatGPT has shown the potential of emerging general artificial intelligence capabilities, as it has demonstrated competent performance across many natural language processing tasks. In this work, we provide first insight into the potential ‘full’ emergence of tasks by broad-data trained foundation models. We approached this from the perspective of natural language tasks in the Affective Computing domain, and chose ChatGPT as exemplary foundation model. To this end, we introduced a framework to evaluate the performance of ChatGPT as a generalist foundation model against specialised models on a total of seven classification tasks from three affective computing problems, namely, personality assessment, sentiment analysis, and suicide tendency assessment.
13.15 – 13.20: Summary and concluding remarks
S3A'21 (2nd March 2021, virtual)
14.30 – 14.35: Welcoming and introduction
14.35 – 15.00: Fabrizio Sebastiani (Italian National Research Council)
Cross-Lingual Sentiment QuantificationSentiment quantification is the task of estimating the relative frequency of sentiment-related classes — such as Positive and Negative — in a set of unlabeled documents. It is an important topic in sentiment analysis, as the study of sentiment-related quantities and trends across a population is often of higher interest than the analysis of individual instances. We propose a method for cross-lingual sentiment quantification, the task of performing sentiment quantification when training documents are available for a source language S but not for the target language T for which sentiment quantification needs to be performed. Cross-lingual sentiment quantification (and cross-lingual text quantification in general) has never been discussed before in the literature; we establish baseline results for the binary case by combining state-of-the-art quantification methods with methods capable of generating cross-lingual vectorial representations of the source and target documents involved.
15.00 – 15.15: Hwee Tou Ng (NUS School of Computing)
Feature Adaptation of a PLM across Domains and Languages for Sentiment ClassificationIn sentiment classification, given a natural language text, we would like to determine whether the document conveys positive, negative, or neutral sentiment. The standard approach to build a sentiment classifier involves supervised training for each individual domain and language, which is time-consuming and not so scalable. In this talk, I will present our recent work on adapting a pre-trained language model XLM-R across different domains and languages. We employ self-training and feature self-distillation for unsupervised domain adaptation. Extensive experiments on two Amazon review datasets on sentiment classification show that our proposed method is effective and outperforms prior state-of-the-art approaches.
15.15 – 15.30: Jing Jiang (SMU School of Information Systems)
Entity-Sensitive Attention and Fusion Network for Entity-Level Multimodal Sentiment AnalysisEntity-level (aka target-dependent) sentiment analysis of social media posts has recently attracted increasing attention, and its goal is to predict the sentiment orientations over individual target entities mentioned in users’ posts. Most existing approaches to this task primarily rely on the textual content, but fail to consider the other important data sources (e.g., images, videos, and user profiles), which can potentially enhance these text-based approaches. Motivated by the observation, we study entity-level multimodal sentiment classification and aim to explore the usefulness of images for entity-level sentiment detection in social media posts. Specifically, we propose an Entity-Sensitive Attention and Fusion Network (ESAFN) which captures the intra-modality dynamics and learns the entity-sensitive visual representation with an entity-oriented visual attention mechanism.
15.30 – 15.45: Soujanya Poria (SUTD Information Systems Technology and Design)
Recognizing Emotion Cause in ConversationsRecognizing the cause behind emotions in text is a fundamental yet under-explored area of research in NLP. Advances in this area hold the potential to improve interpretability and performance in affect-based models. Identifying emotion causes at the utterance level in conversations is particularly challenging due to the intermingling dynamic among the interlocutors. To this end, we introduce the task of recognizing emotion cause in conversations with an accompanying dataset named RECCON. Furthermore, we define different cause types based on the source of the causes and establish strong transformer-based baselines to address two different sub-tasks of RECCON: 1) Causal Span Extraction and 2) Causal Emotion Entailment.
15.45 – 16.00: Jian Su (A*STAR Institute for Infocomm Research)
Gated Lexicon Reader with Contrastive Contextual Co-Attention for Sentiment Analysis
This work proposes a new neural architecture that exploits readily available sentiment lexicon resources. The key idea is that that incorporating a word-level prior can aid in the representation learning process, eventually improving model performance. To this end, our model employs two distinctly unique components, i.e., (1) we introduce a lexicon-driven contextual attention mechanism to imbue lexicon words with long-range contextual information and (2), we introduce a contrastive co-attention mechanism that models contrasting polarities between all positive and negative words in a sentence. Via extensive experiments, we show that our approach outperforms many other neural baselines on sentiment classification tasks on multiple benchmark datasets.
16.00 – 16.15: Frank Xing (NTU School of Computer Science and Engineering)
Financial Sentiment Analysis: An Investigation into Common Mistakes and Silver Bullets
The recent dominance of machine learning-based natural language processing methods has fostered the culture of overemphasizing model accuracies rather than studying the reasons behind their errors. Interpretability, however, is a critical requirement for many downstream AI and NLP applications, e.g., in finance, healthcare, and autonomous driving. This study, instead of proposing any “new model”, investigates the error patterns of some widely acknowledged sentiment analysis methods in the finance domain. We discover that (1) those methods belonging to the same clusters are prone to similar error patterns, and (2) there are six types of linguistic features that are pervasive in the common errors. These findings provide important clues and practical considerations for improving sentiment analysis models for financial applications.
16.15 – 16.30: Zhaoxia Wang (SMU School of Computing and Information Systems)
Multi-Level Fine-Scaled Sentiment Sensing with Ambivalence Handling
Sentiment analysis has become an increasingly important NLP task to help users make sense of what is happening in the Internet blogosphere and it can be useful for companies. However, most existing sentiment analysis techniques are only able to analyze data at the aggregate level, merely providing a binary classification (positive vs. negative), and are not able to generate finer characterizations of sentiments as well as emotions involved. This work describes a new opinion analysis scheme, i.e., a multi-level fine-scaled sentiment sensing with ambivalence handling. The ambivalence handler is presented in detail along with the strength-level tune parameters for analyzing the strength and the fine-scale of both positive or negative sentiments. It is capable of drilling deeper into text in order to reveal multi-level fine-scaled sentiments as well as different types of emotions.
16.30 – 16.45: Haiyun Peng (Alibaba Group Singapore)
Phonetic-Enriched Text Representation for Chinese Sentiment Analysis
The Chinese pronunciation system offers two characteristics that distinguish it from other languages: deep phonemic orthography and intonation variations. In this work, we hypothesize that these two important properties can play a major role in Chinese sentiment analysis. In particular, we propose two effective features to encode phonetic information and, hence, fuse it with textual information. With this hypothesis, we propose Disambiguate Intonation for Sentiment Analysis (DISA), a network that we develop based on the principles of reinforcement learning. DISA disambiguates intonations for each Chinese character (pinyin) and, hence, learns precise phonetic representations. We also fuse phonetic features with textual and visual features to further improve performance.
16.45 – 17.00: Yinping Yang (A*STAR Institute of High Performance Computing)
Global Sentiments Surrounding the COVID-19 Pandemic on Twitter: Analysis of Twitter Trends
With the World Health Organization’s pandemic declaration and government-initiated actions against coronavirus disease (COVID-19), sentiments surrounding COVID-19 have evolved rapidly. This study aimed to examine worldwide trends of four emotions—fear, anger, sadness, and joy—and the narratives underlying those emotions during the COVID-19 pandemic. Public emotions shifted strongly from fear to anger over the course of the pandemic, while sadness and joy also surfaced. Findings from word clouds suggest that fears around shortages of COVID-19 tests and medical supplies became increasingly widespread discussion points. Anger shifted from xenophobia at the beginning of the pandemic to discourse around the stay-at-home notices. Sadness was highlighted by the topics of losing friends and family members, while topics related to joy included words of gratitude and good health.
17.00 – 17.15: Wei Li (NTU School of Computer Science and Engineering)
BiERU: Bidirectional Emotional Recurrent Unit for Conversational Sentiment Analysis
Sentiment analysis in conversations has gained increasing attention in recent years for the growing amount of applications it can serve, e.g., sentiment analysis, recommender systems, and human-robot interaction. The main difference between conversational sentiment analysis and single sentence sentiment analysis is the existence of context information which may influence the sentiment of an utterance in a dialogue. How to effectively encode contextual information in dialogues, however, remains a challenge. Existing approaches employ complicated deep learning structures to distinguish different parties in a conversation and then model the context information. In this work, we propose a fast, compact and parameter-efficient partyignorant framework named bidirectional emotional recurrent unit for conversational sentiment analysis.
17.15 – 17.30: Navonil Majumder (SUTD Information Systems Technology and Design)
MIME: MIMicking Emotions for Empathetic Response Generation
Current approaches to empathetic response generation view the set of emotions expressed in the input text as a flat structure, where all the emotions are treated uniformly. We argue that empathetic responses often mimic the emotion of the user to a varying degree, depending on its positivity or negativity and content. We show that the consideration of these polarity-based emotion clusters and emotional mimicry results in improved empathy and contextual relevance of the response as compared to the state of the art. Also, we introduce stochasticity into the emotion mixture that yields emotionally more varied empathetic responses than the previous work. We demonstrate the importance of these factors to empathetic response generation using both automatic- and human-based evaluations.
17.30 – 17.45: Erik Cambria (NTU School of Computer Science and Engineering)
SenticNet 6: Ensemble Application of Symbolic and Subsymbolic AI for Sentiment Analysis
Deep learning has unlocked new paths towards the emulation of the peculiarly-human capability of learning from examples. While this kind of bottom-up learning works well for tasks such as image classification or object detection, it is not as effective when it comes to natural language processing. Communication is much more than learning a sequence of letters and words: it requires a basic understanding of the world and social norms, cultural awareness, commonsense knowledge, etc.; all things that we mostly learn in a top-down manner. In this work, we integrate top-down and bottom-up learning via an ensemble of symbolic and subsymbolic AI tools, which we apply to the interesting problem of polarity detection from text. In particular, we integrate logical reasoning within deep learning architectures to discover the 200,000 concepts that make up the lastest version of SenticNet.
17.45 – 17.50: Summary and concluding remarks
S3A'19 (8th March 2019, PARKROYAL)
13.00 – 13.15: Welcoming and introduction
13.15 – 14.00: Mike Thelwall (University of Wolverhampton)
Sentiment Analysis is a Big Suitcase
It is necessary to take a holistic approach to sentiment analysis by handling the many subproblems involved in extracting meaning and polarity from text. Although most works approach it as a simple categorization problem, sentiment analysis is actually a suitcase research problem that requires tackling many NLP tasks. As Marvin Minsky would say, the expression “sentiment analysis” itself is a big suitcase (like many others related to affective computing, such as emotion recognition or opinion mining) that all of us use to encapsulate our jumbled idea about how our minds convey emotions and opinions through natural language. We address the composite nature of the problem via a three-layer structure inspired by the jumping NLP curves’ paradigm. In particular, we argue that there are 15 NLP problems that need to be solved to achieve humanlike performance in sentiment analysis.
14.00 – 14.30: Erik Cambria (NTU School of Computer Science and Engineering)
SenticNet 5: Discovering Conceptual Primitives by Means of Context Embeddings
With the recent development of deep learning, research in
AI has gained new vigor and prominence. While machine learning has succeeded in revitalizing many research fields, such as computer vision, speech recognition, and medical diagnosis, we are yet to witness impressive progress in natural language understanding. One of the reasons behind this unmatched expectation is that, while a bottom-up approach is feasible for pattern recognition, reasoning and understanding
often require a top-down approach. To this end, we coupled sub-symbolic and symbolic AI to automatically discover conceptual primitives from text and link them to commonsense concepts and named entities in a new three-level knowledge representation for sentiment analysis, termed SenticNet 5. In particular, we employed recurrent neural networks to infer primitives by lexical substitution and used them for grounding common and commonsense knowledge by means of multi-dimensional scaling.
14.30 – 15.00: Coffee break
15.00 – 15.30: Nuri Kim (NTU Wee Kim Wee School of Communication and Information)
Beyond Rationality: The Role of Anger and Information in Deliberation
The effects of anger and information were experimentally tested in a small group deliberation setting. The participants were randomly assigned to one of four conditions varying in the level of induced anger and amount of information they received. After reading about a controversial local issue, they engaged in a group discussion about the topic. Content analysis revealed each individual’s level of participation. More informed participants expressed more opinions during deliberation than less informed participants. Participants made to feel angry were also more active than those in the control condition, but to a lesser degree than the more informed participants. Structural equation modeling suggested that the effect of anger on postdeliberation argument repertoire and knowledge was more direct, whereas the effect of information was mediated by active participation during deliberation.
15.30 – 16.00: Shirley Ho (NTU Wee Kim Wee School of Communication and Information)
Subjectivity Detection in Nuclear Energy Tweets
Subjectivity detection is an important binary classification task that aims at distinguishing natural language texts as opinionated (positive or negative) and non-opinionated (neutral). We developed and applied recent subjectivity detection techniques to determine subjective and objective tweets towards the hot topic of nuclear energy. This will further help us to detect the presence or absence of social media bias towards Nuclear Energy. In particular, significant network motifs of words and concepts were learned in dynamic Gaussian Bayesian networks, while using Twitter as a source of information. We used reinforcement learning to update each weight based on a probabilistic reward function over all the weights and, hence, to regularize the sentence model. The proposed framework opens new avenues in helping government agencies manage online public opinion to decide and act according to the need of the hour.
16.00 – 17.00: Summary and concluding remarks
S3A'17 (17th February 2017, NTU)
10.00 – 10.15: Welcoming and introduction
10.15 – 11.00: Andrew Ortony (Northwestern University)
The Cognitive Structure of Emotions
What causes us to experience emotions? What makes emotions vary in intensity? How are different emotions related to one another and what are the information processing mechanisms and structures that underlie their elicitation and intensification? In his talk, Prof Ortony will address such questions by presenting a computationally tractable account of the cognitive antecedents of emotions based on three aspects of the world to which agents can react emotionally––events of concern to them, the actions of those they consider responsible for such events, and objects qua objects. He will show how these three classes of reactions lead to three classes of emotions, each based on evaluations in terms of different kinds of knowledge representations. He will also discuss various factors that influence the intensity of emotions while emphasizing the distinction between emotions themselves and the language we use to talk about them.
11.00 – 11.30: Bee Chin Ng (NTU School of Humanities and Social Sciences)
Feeling the Language You Speak
The view that language mediates the world of emotion has been robustly supported in both observations and empirical investigations. The issue of how bilinguals or multilinguals negotiate the emotional worlds of the different languages they speak is beginning to receive attention though the bulk of the studies focus mainly on crosslinguistic comparisons or on monolinguals. There is sufficient evidence, however, to point to bilinguals shifting their sociocultural views and expectations when the language they use changes. This is hardly surprising but what remains to be explored is precisely how these views change and whether these changes can be predicted by our current knowledge of emotion research about existing languages. In this talk I will present the findings from my current study of an emotion corpus in Mandarin Chinese as well as a crosslinguistic comparison of four emotion domains (Anger, Pride, Guilt and Shame). The findings indicate strong language effects and despite fairly homogenous cultural experience, language use and exposure play a big role in shaping Singaporean bilinguals’ use and understanding of emotion words. This key aspect of the findings challenges the common practice to see same language pair bilinguals as a homogenous bilingual group.
11.30 – 12.00: Jing Jiang (SMU School of Information Systems)
Learning Sentence Embeddings for Cross-Domain Sentiment Classification
Recent years have witnessed the promising results of applying neural network models in many natural language processing tasks, including sentiment analysis and opinion mining. However, as with any supervised learning method, neural network models cannot work well when the training data comes from a different domain than the test data. In this talk, I will present two recent pieces of work that borrow labels of auxiliary tasks to improve the cross-domain performance for sentiment classification and opinion target extraction. In both studies the auxiliary labels are used to improve the learning of the hidden representations of text in a multi-layer neural network.
12.00 – 12.30: Andrea Nanetti (NTU School of Art, Design and Media)
One Belt, One Road, One Sentiment?
One Belt, One Road, One Sentiment? is a project in collaboration with Prof Andrea Nanetti, from NTU ADM, which aims to visualize the sentiment of the world towards President Xi's One Belt One Road initiative. Such an initiative is a $4-trillion development strategy and framework that focuses on connectivity and cooperation among 60 countries primarily between China and the rest of Eurasia and consists of two main components: the land-based Silk Road Economic Belt and ocean-going Maritime Silk Road. One Belt, One Road, One Sentiment? aims to collect and analyze the reactions of the different countries involved in President Xi's initiative in real-time. Many economies, in fact, are affected by the initiative, which has been welcomed by some countries but contrasted by some others, e.g., the supporters of trading arrangements such as the Trans-Pacific Partnership and the Transatlantic Trade and Investment Partnership. The project employs SenticNet technologies to collect and analyze news and social media in many different languages from across the globe and, hence, visualize the real-time sentiment of the world towards the One Belt One Road initiative in real-time in a 3D dome.
12.30 – 13.30: Lunch break
13.30 – 14.00: Vibeke Sorensen (NTU School of Art, Design and Media)
Mood of the Planet: Challenging Visions of Big Data in the Arts
Mood of the Planet is an interactive physical-digital sculpture that has as its center-piece a large ‘arch’ or ‘doorway’ that emits colored light and sound as a form of visualization and sonification of the changing, live emotions expressed by people all around the Earth. It is
the product of several disciplines, including the arts, computer science, linguistics and psychology. In particular, we use artificial intelligence to collect
and analyze social media data and extract emotions from these using a braininspired and psychologically-motivated emotion categorization model.
Such emotions are then translated into colors and sounds that the audience
can experience while passing through the arch. Feedback from the
audience proved the Mood of the Planet to provide a more accurate, personal
and tangible experience about the data-emotions dichotomy.
14.00 – 14.30: Erik Cambria (NTU School of Computer Science and Engineering)
SenticNet 4
In this talk, I am going to reveal some of the magic behind Mood of the Planet and One Belt, One Road, One Sentiment? by presenting the latest version of SenticNet, a commonsense knowledge base for concept-level sentiment analysis. SenticNet encodes affective information in terms of semantics and sentics, i.e., the denotative and connotative information commonly associated with real-world objects, actions, events, and people. SenticNet steps away from blindly using keywords and word co-occurrence counts, and instead relies on the implicit meaning associated with commonsense concepts. Superior to purely syntactic techniques, SenticNet can detect subtly expressed sentiments by enabling the analysis of multiword expressions that do not explicitly convey emotion, but are instead related to concepts that do so.
14.30 – 15.00: Chris Khoo (NTU Wee Kim Wee School of Communication and Information)
Lexicon-Based Sentiment Analysis: Comparative Evaluation of Six Sentiment Lexicons
This talk describes a general-purpose sentiment lexicon called WKWSCI Sentiment Lexicon, and compares it with five existing lexicons: Hu & Liu Opinion Lexicon, MPQA Subjectivity Lexicon, General Inquirer, NRC Word-Sentiment Association Lexicon and SO-CAL lexicon. The effectiveness of the sentiment lexicons for sentiment categorization at the document-level and sentence-level was evaluated using an Amazon product review dataset and a news headlines dataset. WKWSCI, MPQA, Hu & Liu and SO-CAL lexicons are equally good for product review sentiment categorization, obtaining accuracy rates of 75% to 77% when appropriate weights are used for different categories of sentiment words. However, when a training corpus is not available, Hu & Liu obtained the best accuracy with a simple-minded approach of counting positive and negative words, for both document-level and sentence-level sentiment categorization. The WKWSCI lexicon obtained the best accuracy of 69% on the news headlines sentiment categorization task, and the sentiment strength values obtained a Pearson correlation of 0.57 with human assigned sentiment values.
15.00 – 15.30: Gao Cong (NTU School of Computer Science and Engineering)
A Unified Model for User Preference Analysis in Geo-tagged Reviews
Massive amounts of geo-textual data that contain both geospatial and textual content are being generated at an unprecedented scale from social media websites. Example user generated geo-textual content includes geo-tagged micro-blogs, photos with both tags and geo-locations in social photo sharing websites, as well as points of interest (POIs) and check-in information in location-based social networks. This talk presents a study of user preferences to POIs based on geo-tagged reviews. We study two types of user preferences to POIs: topical-region preference and category aware topical-aspect preference. We propose a unified probabilistic model to capture these two preferences simultaneously.
In addition, our model is capable of capturing the interaction of different factors, including topical aspect, sentiment, and spatial information. The model can be used in a number of applications, such as POI recommendation and user recommendation, among others. In addition, the model enables us to investigate whether people like an aspect of a POI or whether people like a topical aspect of some type of POIs (e.g., bars) in a region, which offer explanation for recommendations.
15.30 – 16.00: Coffee break
16.00 – 16.20: Aixin Sun (NTU School of Computer Science and Engineering)
Domain-Specific Named Entity Recognition from Social Media
Named entity recognition (NER) is an important task in text analysis. NER from social media text is even more challenging because of the informal writing style and the lack of context in interpreting the content. In this talk, I will report two case studies on domain specific NER from social media. One study is to recognize mobile phone names from online forum and the other is to recognize fine-grained locations (e.g., restaurants, landmarks, and shopping malls) from tweets. For both tasks, we propose to build a dictionary in user language with noisy terms that might be a named entity or a part of a named entity. A classifier is then built to recognize the named entities and then link to their formal names. Our experiments on manually annotated data show promising results on both tasks.
16.20 – 16.40: Danyuan Ho (NTU Temasek Laboratories)
Singlish SenticNet
Singlish SenticNet is a concept-level resource for sentiment analysis in Singlish that provides the semantics and sentics (denotative and connotative information) associated with more than 5000 words and multiword expressions. These concepts are crowdsourced (e.g., through games) and encoded redundantly at three levels, namely: as a semantic network, as a matrix and as a vector space. Each representation is useful for a different kind of reasoning: the semantic network specifies the relationships between concepts and, hence, it is useful for tasks such as question answering; the matrix allows for the inference of new pieces of commonsense knowledge based on shared semantic features; finally, the vector space is a powerful tool for analogical reasoning. Singlish SenticNet is generated by the joint use of all three representations: this superior kind of reasoning, termed sentic panalogy, ensures that both the accuracy and efficiency of Singlish SenticNet are high by making the most of each representation at different times. In problem-solving situations, in fact, several analogous representations of the same problem should be maintained in parallel while trying to solve it so that, when problem-solving begins to fail while using one representation, the system can switch to one of the others.
16.40 – 17.00: Stefan Winkler (ADSC)
Fine-grained Emotion Analysis From Facial Expressions
Emotions are often conveyed by facial expressions. Contrary to most existing approaches in computer vision, we avoid the classification of emotions into a few predefined categories, like happy, sad, or surprised, and instead follow a dimensional paradigm as represented by the circumplex model of emotions. We cast the problem of emotion estimation as a regression problem. Based on the tracking of facial landmark points and relevant geometrical features, we directly estimate arousal, valence, and intensity of emotion. Our model is trained using a large database of face images from psychophysical validation studies. This approach more accurately encompasses the wide range of facial expressions, and is able to capture even subtle variations in emotion and intensity. We discuss the benefits and challenges of our method, and also present some of its applications.
17.00 – 17.20: Iti Chaturvedi (NTU School of Computer Science and Engineering)
Multiple Kernel Learning for Multimodal Sentiment Analysis
Technology has enabled anyone with an Internet
connection to easily create and share their ideas, opinions and
content with millions of other people around the world. Much
of the content being posted and consumed online is multimodal.
With billions of phones, tablets and PCs shipping today with
built-in cameras and a host of new video-equipped wearables like
Google Glass on the horizon, the amount of video on the Internet
will only continue to increase. It has become increasingly difficult
for researchers to keep up with this deluge of multimodal content,
let alone organize or make sense of it. Mining useful knowledge
from video is a critical need that will grow exponentially, in
pace with the global growth of content. This is particularly
important in sentiment analysis, as both service and product
reviews are gradually shifting from unimodal to multimodal.
We present a novel method to extract features from visual and
textual modalities using deep convolutional neural networks. By
feeding such features to a multiple kernel learning classifier,
we significantly outperform the state of the art of multimodal sentiment analysis on different datasets.
17.20 – 18.00: Summary and concluding remarks
S3A'15 (6th February 2015, NTU)
10.00 – 10.15: Welcoming and introduction
10.15 – 11.00: Amit Sheth (Ohio Center of Excellence in Knowledge-enabled Computing)
Citizen Sensor Data Mining, Social Media Analytics and Applications
With the rapid rise in the popularity of social media (1B+ Facebook users, 200M+ twitter users), and near ubiquitous mobile access (4+ billion actively-used mobile phones), the sharing of observations and opinions has become common-place (500M+ tweets a day). This has given us an unprecedented access to the pulse of a populace and the ability to perform analytics on social data to support a variety of socially intelligent applications -- be it for brand tracking and management, crisis coordination, organizing revolutions or promoting social development in underdeveloped and developing countries. I will review: 1) understanding and analysis of informal text, esp. microblogs (e.g., issues of cultural entity extraction and role of semantic/background knowledge enhanced techniques), and 2) how we built Twitris, a comprehensive social media analytics (social intelligence) platform. I will describe the analysis capabilities along three dimensions:
spatio-temporal-thematic,
people-content-network, and
sentiment-emption-intent.
I will couple technical insights with identification of computational techniques and real-world examples using live demos of Twitris (http://analysis.knoesis.org)
11.00 – 11.45: Tomoko Ohkuma (Fuji-Xerox)
Sentiment Analysis and User Profiling for SNS Text
The NLP team in the Communication Technology Laboratory is working on research and development of information extraction from SNS text. In this presentation, we introduce research activities about sentiment analysis and user profiling for applications like social listening, reputation management, and marketing.
Topics that will be presented are 1) a report of SemEval-2014, 2) sentiment analysis using WSD, 3) targeted sentiment using topic modeling, 4) user gender inference using text and image processing.
At the end of this presentation, we talk about a new joint research project that just started between NTU and Fuji Xerox in this February.
11.45 – 12.15: Waifong Boh (NTU Nanyang Business School)
A Temporal Study of the Effects of Online Opinions: Information Sources Matter
This study examines when and why online comments from different sources and platforms influence a movie's box office receipts over time. We tracked over 1,500 sources of online expert and consumer reviews for cinematic movies released for an entire year and continuously monitored major social media sites (e.g. Twitter and Plurk) for comments. We text-mined the comments to elucidate the sentiments and analyzed the data. Premised on the argument that greater uncertainty exists at the beginning of a movie's release, we hypothesized and found that expert reviews, and the valence and volume of comments from pull-based platforms like forums have a significant influence on early box office receipts. In contrast, the valence and volume of comments from push-based platforms like microblogs have a significant influence on later box office receipts, as they serve a reminder rather than an informational role with the decreased uncertainty in these later stages. Our research demonstrates that online opinions are not always persuasive and useful, and our findings provide insights into when consumers are likely to pay attention to which types of online opinions.
12.15 – 12.45: Feida Zhu (SMU School of Information Systems)
Social Media Mining and Analysis for Financial InnovationThe recent blossom of social network services has provided everyone with an unprecedented level of ease and fun of sharing information of all sorts. These public social data therefore reveal a surprisingly large amount of information about an individual which is otherwise unavailable. The business, consumer and social insights attainable from this big and dynamic social data are critically important and immensely valuable in a wide range of applications for both private and public sectors. In particular, there has been a growing interest in harnessing social media data for financial innovation. In this talk, we will explore some recent advances along this direction including personal credit scoring, risk management and customer acquisition.
12.45 – 14.00: Lunch break
14.00 – 14.30: Chris Khoo (NTU Wee Kim Wee School of Communication and Information)
Comparison of Lexical Resources for Sentiment Analysis
This work sets out a detailed comparison of sentiment lexica (General inquirer, MPQA and Hu & Liu) with WKWSCI lexicon. WKWSCI lexicon contains human annotated words with semantic orientation (polarity and strength). The presentation will provide an overview of the coverage of WKWSCI lexicon, overlap and consistency with other lexicons. We also show lexicon performance in product reviews dataset using bag of words approach.
14.30 – 15.00: Elvis Albertus Bin Toni (NTU School of Humanities and Social Sciences)
Linguistic Expression of Emotions in Lamaholot Language
This study observed the syntactical differences across dialects, metaphors, and borrowing from and/or mixing with other language for linguistic expression of emotions in Lamaholot language. It displays several findings that there are two distinctive syntactical features i.e. the existence of pronoun subject in the expression of emotions and the use of single combination of morphemes across three investigated dialects (Nusa Tadon, Lewo Tobi, and Lewolema). That a metaphor is a vehicle for expression of emotion attested in the three dialects. That ‘One-k’/my heart as a feature of expression of emotion in Lamaholot is shared among the dialects. That borrowing from and/or mixing with Bahasa Indonesia when expressing emotion is common.
15.00 – 15.30: Iti Chaturvedi (NTU School of Computer Science and Engineering)
Deep Recurrent Neural Networks for Sentiment AnalysisThe rise in social media such as blogs and networking websites has resulted in a surge of research in sentiment classification, which aims to determine the judgment of a writer with respect to a given topic based on a given textural comment. The objective is to classify the sentiment polarity of a tweet as positive, negative, or neutral. We propose use of a deep neural network to automatically extract sentiment specific word embedding from tweets. To capture loops and higher-order dependencies in a sequence of words we use Gaussian Bayesian networks. Low dimensional statistically significant word-structures called motifs are extracted from a variety of sources of data.
15.30 – 16.00: Francis Bond (NTU School of Humanities and Social Sciences)
Multi-Lingual Semantic ProcessingWith physical barriers to information access decreasing, lack of understanding become the greatest impediment to communication. Research on deep linguistic analysis allows us to abstract away from language particular syntactic phenomena to a uniform panlingual semantic representation. By linking this to WordNet, we can take advantage of a wide variety of linked open data, including sentiment and apply it to hundreds of languages.
16.00 – 16.30: Erik Cambria (NTU School of Computer Science and Engineering)
Sentic Patterns
Sentic patterns merge linguistics, commonsense computing, and machine learning for improving the accuracy of sentiment-analysis tasks such as polarity detection. Sentic patterns allow sentiments to flow from concept to concept based on the dependency relation of the input sentence, like in an electronic circuit where sentiment words are sources while other words are elements, e.g., VERY is an amplifier, NOT is a logical complement, RATHER is a resistor, BUT is an OR-like element that gives preference to one of its inputs. This way, sentic patterns achieve a better understanding of the contextual role of each concept within the sentence and, hence, obtain a polarity detection accuracy that outperforms state-of-the-art statistical methods.
16.30 – 17.00: Summary and concluding remarks
S3A'13 (1st November 2013, NTU)
13.00 – 13.10: Welcoming and introduction
13.10 – 13.30: Grégoire Winterstein (Hong Kong Institute of Education)
Argumentative Operators and Sentiment AnalysisI will provide a brief characterization of the notion of argumentation as it is understood in psychology and linguistics. I will then proceed to show how some linguistic items can best be described in argumentative terms. I will focus on the contributions of 'only', and 'almost'. In a second part I will underline the possible uses of argumentative theories for sentiment analysis and the insights argumentative theories can gather from the output of sentiment analysis models.
13.30 – 13.50: Hai Zhen (NTU School of Computer Science and Engineering)
Product Review MiningMy talk will focus on product review mining, as briefly summarized below
1. Introduction to review mining (opinion mining, sentiment analysis): background, motivation, introduction
2. Review mining at document (review), sentence, or phrase level
3. Feature-level review mining
3.1 feature extraction
3.1.1 explicit feature
3.1.2 implicit feature
3.2 opinion word identification and sentiment polarity classification
3.3 summarization
4. Aspect-based review mining (mainly discuss Topic Models)
4.1 aspect detection
4.1 sentiment prediction
5. review helpfulness prediction and review selection
6. Experiments
7. Conclusion
13.50 – 14.10: Lin Qiu (NTU School of Humanities and Social Sciences)
Personality Analysis over Twitter
Microblogging services such as Twitter have become increasingly popular in recent years. However, little is known about how personality is manifested and perceived in microblogs. In this study, we measured the Big Five personality traits of 142 participants and collected their tweets over a 1-month period. Extraversion, agreeableness, openness, and neuroticism were associated with specific linguistic markers, suggesting that personality manifests in microblogs. Meanwhile, eight observers rated the participants’ personality on the basis of their tweets. Results showed that observers relied on specific linguistic cues when making judgments, and could only judge agreeableness and neuroticism accurately. This study provides new empirical evidence of personality expression in naturalistic settings, and points to the potential of utilizing social media for personality research.
14.10 – 14.30: Chris Khoo (NTU Wee Kim Wee School of Communication and Information)
Sentiment Analysis of Movie Reviews, Drug Reviews and Political NewsThe talk summarizes 3 studies on the sentiment analysis of movie reviews, drug reviews and political news. The first study analyzed the differences in sentiment expressions used in movie reviews from four Web genres—blog postings, discussion board threads, user reviews, and reviews by movie critics. Sentiment analysis of movie reviews was performed at the clause level to identify the sentiment orientation and strength towards different aspects of a movie. A method was developed to compute the overall sentiment of a clause based on the sentiment scores of individual words, taken from sentiment lexicons. A visual interface was developed to explore the extracted sentiments. More recently, a similar sentiment analysis approach was applied to drug reviews. The third study was a case study of applying the Appraisal Theory developed by linguists to analyze political news articles.
14.30 – 14.50: Coffee break
14.50 – 15.10: Bai Lin (NTU School of Humanities and Social Sciences)
Communicating Emotions across Cultures
In our increasingly interconnected world, how to communicate across different cultures has become more critical. However, successful communications are always hindered by differences between languages and cultures, and such difficulties become even more obvious when it comes to more personal and emotional topics. How do people from diverse culture backgrounds communicate their emotions? In what ways does an expression of emotion vary across culture? How bilinguals meet with the challenges of cultural or linguistic specificities of their two languages? Can these cultural knowledge of emotion expression be taught?
15.10 – 15.30: Guang-Bin Huang (NTU School of Electrical & Electronic Engineering)
Representational Learning with Extreme Learning Machine for Big Data
Neural networks (NN) and support vector machines (SVM) play key roles in machine learning and data analysis in the past 2-3 decades. However, it is known that these popular learning techniques face some challenging issues such as: intensive human intervene, slow learning speed, poor learning scalability. Extreme Learning Machines (ELM) not only learn up to tens of thousands faster than NN and SVMs, but also provide unified implementation for regression, binary and multi-class applications. This talk will give a brief introduction to ELM history and some of its successful applications. This talk will further address three issues: i) why NN and SVM/LS-SVM may only produce suboptimal solutions to ELM; ii) why ELM may outperform Deep Learning in both learning accuracy and learning speed; and iii) why ELM could be a biological inspired learning technique and why ELM is closer to animal brains.
15.30 – 15.50: Francis Bond (NTU School of Humanities and Social Sciences)
Uniform Cross-lingual Sentiment analysis with WordNets
Semantically annotated corpora play an
important role in natural language processing.
This talk presents the results
of a pilot study on building a sense-tagged
parallel corpus, part of ongoing construction
of aligned corpora for four languages
(English, Chinese, Japanese, and Indonesian)
in four domains (story, essay, news,
and tourism) from the NTU-Multilingual
Corpus. Each subcorpus is first sensetagged
using a WordNet and then these
synsets are linked. Upon the completion
of this project, all annotated corpora will
be made freely available. The multilingual
corpora are designed to not only provide
data for NLP tasks like machine translation,
but also to contribute to the study
of translation shift and bilingual lexicography
as well as the improvement of monolingual
WordNets.
15.50 – 16.10: Erik Cambria (NUS Temasek Labs)
Jumping NLP Curves
Natural language processing (NLP) is a theory-motivated
range of computational techniques for the automatic analysis and representation of human language. NLP research has evolved from the era
of punch cards and batch processing (in which the analysis of a sentence could take up to 7 minutes) to the era of Google and the likes of it (in which millions of webpages can be processed in less than a second). This presentation draws on recent developments in
NLP research to look at the past, present, and future of NLP technology in a new light. Borrowing the paradigm of ‘jumping curves’ from the field of business management and marketing prediction, this talk reinterprets the evolution of NLP research as
the intersection of three overlapping curves-namely Syntactics, Semantics, and Pragmatics Curves- which will eventually lead NLP research to evolve
into natural language understanding.
16.10 – 16.30: Summary and concluding remarks